Early machine learning prediction of hospitalized patients at low risk of respiratory deterioration or mortality in community-acquired pneumonia: Derivation and validation of a multivariable model
DOI:
https://doi.org/10.17305/bb.2023.9754Keywords:
Community-acquired pneumonia (CAP), machine learning, predictive modeling, advanced respiratory support, mortalityAbstract
Current prognostic tools for pneumonia predominantly focus on mortality, often neglecting other crucial outcomes such as the need for advanced respiratory support. The objective of this study was to develop and validate a tool that predicts the early risk of non-occurrence of respiratory deterioration or mortality. We conducted a single-center, retrospective cohort study involving hospitalized adult patients with community-acquired pneumonia (CAP) and acute hypoxic respiratory failure from January 2009 to December 2019 (n = 4379). We employed the gradient boosting machine (GBM) learning to create a model that estimates the likelihood of patients requiring advanced respiratory support (high flow nasal cannula [HFNC], non-invasive mechanical ventilation [NIMV], and invasive mechanical ventilation [IMV]) or facing mortality during hospitalization. This model utilized readily available data including demographic, physiologic, and laboratory data, sourced from electronic health records and obtained within the first six hours of admission. Out of the cohort, 890 patients (25.2%) either required advanced respiratory support or died during their hospital stay. Our predictive model displayed superior discrimination and higher sensitivity (cross-validation C-statistic = 0.71; specificity = 0.56; sensitivity = 0.72) compared to the pneumonia severity index (PSI) (C-statistic = 0.65; specificity = 0.91; sensitivity = 0.24; P value < 0.001), while maintaining a negative predictive value (NPV) of approximately 0.85. These data demonstrate that our machine learning model predicted the non-occurrence of respiratory deterioration or mortality among hospitalized CAP patients more accurately than the PSI. The enhanced sensitivity of this model holds potential for reliably excluding low-risk patients from pneumonia clinical trials.
Citations
Downloads
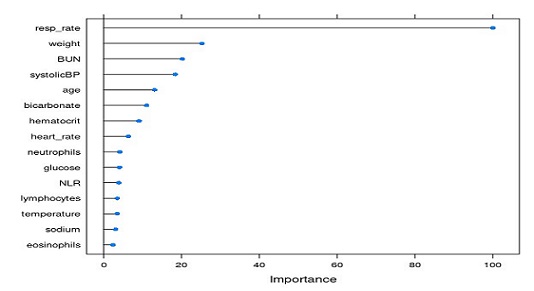
Downloads
Published
Issue
Section
Categories
License
Copyright (c) 2023 Yewande E. Odeyemi, Amos Lal, Erin F. Barreto, Allison M. LeMahieu, Hemang Yadav, Ognjen Gajic, Phillip Schulte

This work is licensed under a Creative Commons Attribution 4.0 International License.
How to Cite
Accepted 2023-09-26
Published 2024-03-11