Detecting cell types and densities in the tumor microenvironment improves prognostic risk assessment for breast cancer
DOI:
https://doi.org/10.17305/bb.2024.10974Keywords:
Tumor microenvironment, transfer learning, breast cancer, artificial intelligence, deep convolutional neural networkAbstract
A comprehensive evaluation of the relationship between the densities of various cell types in the breast cancer tumor microenvironment and patient prognosis is currently lacking. Additionally, the absence of a large patch-level whole slide imaging (WSI) dataset of breast cancer with annotated cell types hinders the ability of artificial intelligence to evaluate cell density in breast cancer WSI. We first employed Lasso-Cox regression to build a breast cancer prognosis assessment model based on cell density in a population study. Pathology experts manually annotated a dataset containing over 70,000 patches and used transfer learning based on ResNet152 to develop an artificial intelligence model for identifying different cell types in these patches. The results showed that significant prognostic differences were observed among breast cancer patients stratified by cell density score (P = 0.0018), with the cell density score identified as an independent prognostic factor for breast cancer patients (P < 0.05). In the validation cohort, the predictive performance for overall survival (OS) was satisfactory, with area under the curve (AUC) values of 0.893 (OS) at 1-year, 0.823 (OS) at 3-year, and 0.861 (OS) at 5-year intervals. We trained a robust model based on ResNet152, achieving over 99% classification accuracy for different cell types in patches. These achievements offer new public resources and tools for personalized treatment and prognosis assessment.
Citations
Downloads
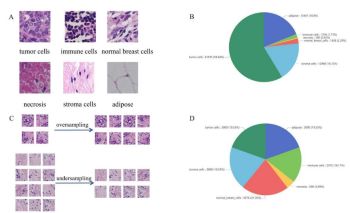
Downloads
Published
Issue
Section
License
Copyright (c) 2024 Pu Liu, Xueli Zhang, Wenwen Wang, Yunping Zhu, Yongfang Xie, Yanhong Tai, Jie Ma

This work is licensed under a Creative Commons Attribution 4.0 International License.