Development and evaluation of interpretable machine learning regressors for predicting femoral neck bone mineral density in elderly men using NHANES data
DOI:
https://doi.org/10.17305/bb.2024.10725Keywords:
Femoral neck bone mineral density, osteoporotic femoral neck fractures, NHANES, machine learning, random forest regressorAbstract
Osteoporotic femoral neck fractures (OFNFs) pose a significant orthopedic challenge in the elderly population, accounting for up to 40% of all osteoporotic fractures and leading to considerable health deterioration and increased mortality. In addressing the critical need for early identification of osteoporosis through routine screening of femoral neck bone mineral density (FNBMD), this study developed a user-friendly prediction model aimed at men aged 50 years and older, a demographic often overlooked in osteoporosis screening. Utilizing data from the National Health and Nutrition Examination Survey (NHANES), the study involved outlier detection and handling, missing value imputation via the K nearest neighbor (KNN) algorithm, and data normalization and encoding. The dataset was split into training and test sets with a 7:3 ratio, followed by feature screening through the least absolute shrinkage and selection operator (LASSO) and the Boruta algorithm. Eight different machine learning algorithms were then employed to construct predictive models, with their performance evaluated through a comprehensive metric suite. The random forest regressor (RFR) emerged as the most effective model, characterized by key predictors such as age, body mass index (BMI), poverty income ratio (PIR), serum calcium, and race, achieving a coefficient of determination (R²) of 0.218 and maintaining robustness in sensitivity analyses. Notably, excluding race from the model resulted in sustained high performance, underscoring the model’s adaptability. Interpretations using Shapley additive explanations (SHAP) highlighted the influence of each feature on FNBMD. These findings indicate that our predictive model effectively aids in the early detection of osteoporosis, potentially reducing the incidence of OFNFs in this high-risk population.
Citations
Downloads
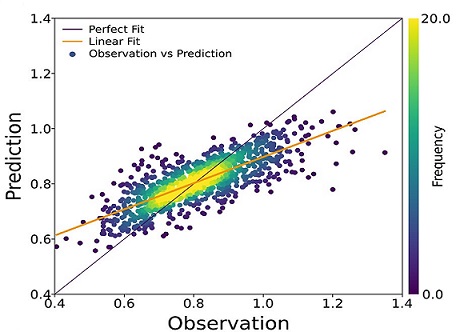
Downloads
Published
Data Availability Statement
The datasets analyzed during the current study are available on the National Health and Nutrition Examination Survey (NHANES) website: https://www.cdc.gov/nchs/index.htm.
Issue
Section
Categories
License
Copyright (c) 2024 Wen He, Song Chen, Xianghong Fu, Licong Xu, Jun Xie, Jinxing Wan

This work is licensed under a Creative Commons Attribution 4.0 International License.